Generative AI is at the forefront of artificial intelligence research and application, opening new horizons in creativity, design, and problem-solving. This cutting-edge technology leverages advanced algorithms to generate text, images, music, and even complex models, mimicking human creativity and intelligence. In this blog, we will explore what generative AI is, how it works, its applications across various industries, and the future possibilities it holds. Forget writer’s block! “Generative AI, Unleashing Creativity with Machine Learning” explores how AI can become your next artistic collaborator.
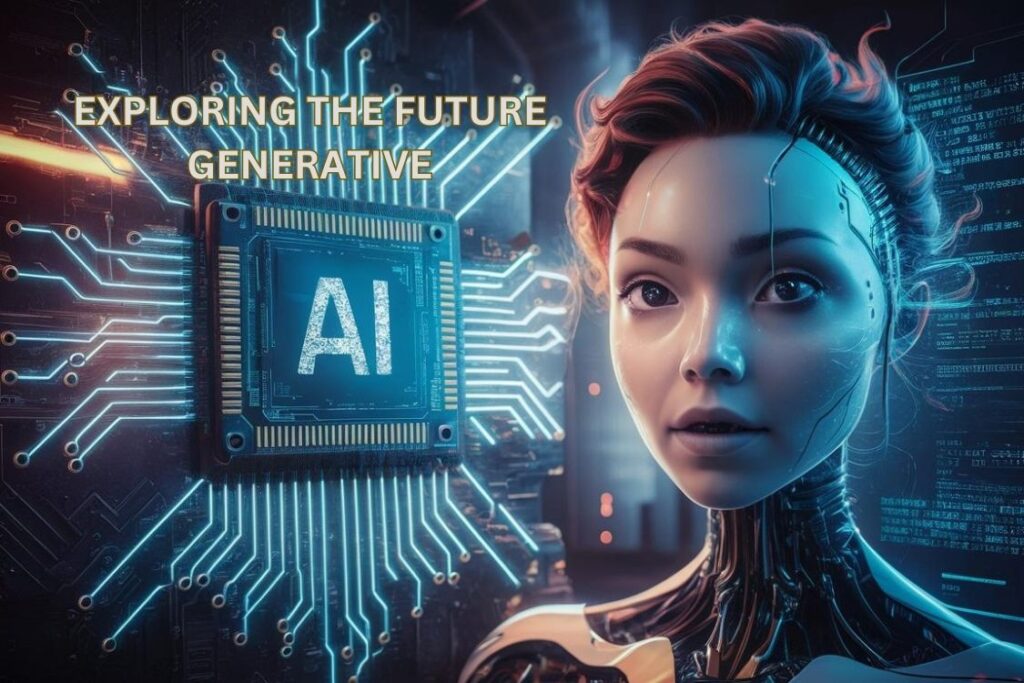
What is Generative AI?
Generative AI refers to a subset of artificial intelligence that uses machine learning models to create new content. Unlike traditional AI, which focuses on analysis and prediction, generative AI is designed to produce novel outputs. These outputs can range from realistic images and human-like text to original music compositions and innovative product designs.
How Does Generative AI Work?
- Generative Adversarial Networks (GANs): GANs consist of two neural networks, the generator and the discriminator, which work in tandem. The generator creates new data instances, while the discriminator evaluates them for authenticity. Through this adversarial process, the generator improves over time, producing increasingly realistic outputs.
- Variational Autoencoders (VAEs): VAEs encode input data into a latent space and then decode it to generate new data. This process allows VAEs to produce diverse and unique outputs while maintaining the general characteristics of the input data.
- Recurrent Neural Networks (RNNs): RNNs generate sequences (e.g., text, music) by predicting the next element based on the previous ones.
Applications of Generative AI
- Art and Design: Artists and designers use generative AI to create original artworks, graphics, and designs. AI-generated art is gaining popularity in galleries and online platforms, offering new forms of creative expression.
- Content Creation: Generative AI can write articles, generate social media posts, and produce marketing copy. Tools like GPT-4 (the model behind this blog) can generate human-like text, making content creation faster and more efficient.
- Gaming and Entertainment: In the gaming industry, generative AI is used to create characters, environments, and storylines, enhancing the gaming experience. In music, AI can compose original pieces, providing new opportunities for musicians and composers.
- Healthcare: Generative AI aids in drug discovery by predicting molecular structures and generating potential compounds. It also helps in creating synthetic medical data for research and training purposes.
- Fashion: Designers use generative AI to explore new styles and patterns, allowing for innovative fashion designs and personalized clothing recommendations.
- Finance: In finance, generative AI can simulate market scenarios, helping in risk assessment and strategic planning. It also assists in generating realistic synthetic data for algorithm training.
- Data Augmentation: Generative models enhance datasets by creating synthetic examples, improving model performance.
Benefits of Generative AI
- Enhanced Creativity: Generative AI expands the creative capabilities of individuals and organizations, enabling the production of unique and innovative content.
- Efficiency and Productivity: Automating content creation and design processes saves time and resources, allowing professionals to focus on higher-level tasks.
- Personalization: AI-generated content can be tailored to individual preferences, providing personalized experiences in marketing, entertainment, and product recommendations.
- Innovation: By generating novel ideas and solutions, generative AI drives innovation across industries, from healthcare to finance.
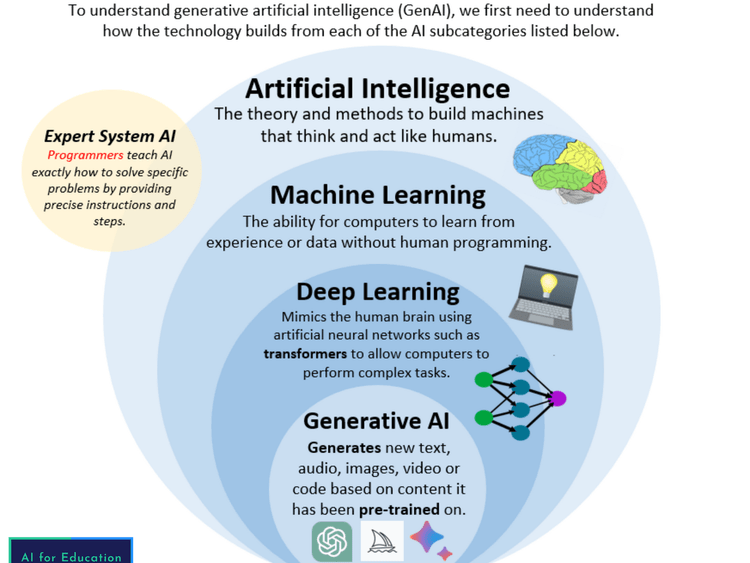
Challenges and Ethical Considerations
Despite its potential, generative AI also poses challenges and ethical concerns:
- Quality Control: Ensuring the quality and accuracy of AI-generated content can be difficult, requiring human oversight and intervention.
- Bias and Fairness: Generative AI models can perpetuate existing biases in training data, leading to biased outputs. Addressing fairness and inclusivity is crucial.
- Intellectual Property: The ownership of AI-generated content raises legal questions about intellectual property rights and authorship.
- Misinformation: The ability of generative AI to create realistic fake content poses risks related to misinformation and deepfakes.
The Future of Generative AI
The future of generative AI is promising, with ongoing research and advancements poised to enhance its capabilities and applications. Key areas of development include:
- Improved Models: Continued advancements in neural network architectures and training techniques will lead to more sophisticated and reliable generative AI models.
- Ethical AI: Efforts to address bias, fairness, and transparency will ensure that generative AI is used responsibly and ethically.
- Cross-Industry Integration: Generative AI will increasingly be integrated across industries, driving innovation and transforming business processes.
- Human-AI Collaboration: The synergy between human creativity and AI capabilities will unlock new possibilities, allowing for collaborative creation and problem-solving.
Conclusion
Generative AI is revolutionizing the way we create, innovate, and solve problems. By harnessing the power of advanced algorithms, generative AI opens up new avenues for artistic expression, content creation, and industry transformation. As we navigate the challenges and ethical considerations, the future of generative AI promises to be a dynamic and transformative force in our increasingly digital world. Whether you’re an artist, entrepreneur, researcher, or simply curious about the future, generative AI offers exciting possibilities that are just beginning to unfold.
TO LEARN MORE
- Embracing the Future of Artificial Intelligence – Krutrim AI
- AI-Powered CRM and Automation Tools
- Artificial Rain: Enhancing Precipitation Through Cloud Seeding
- BrainBridge: Pioneering the Future of Head Transplants
- Neuralink: Bridging the gap between brain and computer
- EctoLife: The World’s First Artificial Womb Facility
- Mobile Gaming: Trends Shaping the Future
- The 10 Most Popular YouTube Channels
- Beyond the Brochure: VR & AR Reimagine Hospitality
- VR & AR: Reshaping industries with immersive experiences
- The Future of Traffic Signal Systems With AI
- Ilya Sutskever Has a New Plan for Safe Superintelligence
- Claude 3.5 Sonnet: Frontier Intelligence at 2x the Speed
- Understanding Docker: Software Development and Deployment
- G-Assist: NVIDIA’s Gaming Assistance
- Google DeepMind’s V2A
Frequently Asked Questions (FAQs)
Generative AI refers to a subset of artificial intelligence that focuses on creating new content, such as text, images, music, or even entire designs, by learning patterns from existing data. It uses advanced algorithms to generate outputs that mimic human creativity and intelligence.
Generative AI works using neural networks, particularly Generative Adversarial Networks (GANs) and Variational Autoencoders (VAEs). GANs consist of a generator that creates data and a discriminator that evaluates it, improving the generator’s output over time. VAEs encode data into a latent space and decode it to generate new content, maintaining the essence of the input data.
Generative AI is a powerful tool within the AI landscape. It allows for creativity and novel content generation, making it useful for various applications. As generative AI technologies continue to evolve, they will play an essential role in shaping our interactions with information, from language and images to video and audio.
Generative AI can accelerate product development, enhance customer experiences, and improve employee productivity. However, the specific benefits depend on the use case. It’s essential to recognize that generative AI models are prediction engines, and their outputs can vary in response to the same prompt. Human validation remains crucial to ensure accuracy and mitigate biases.
Generative AI models can produce inaccurate or biased content. Ensuring proper validation and addressing potential ethical concerns are essential steps in using generative AI effectively.
Thank you for your sharing. I am worried that I lack creative ideas. It is your article that makes me full of hope. Thank you. But, I have a question, can you help me?